By Susan Price
John Spinosa ’80 wanted to try a new approach. Before he’d arrived at the San Diego Blood Bank in the summer of 2017, Spinosa had co-founded a biotech company. Now, as the pathologist and chief medical officer began digging into the blood bank’s fundamental challenge—how to meet the constant demand for multiple varieties of blood from a supply dependent on a largely unpredictable pool of volunteer donors— he turned to one of an entrepreneur’s favorite tools: data.
“I looked at things in a new way for them, thinking about problems as I had at the start-up,” Spinosa said.
The SDBB had been gathering information about donors and donations for about a decade, with spreadsheets listing donors’ ages, hometowns, blood types, and the like. But putting that data to use was another issue. Spinosa knew that machine learning— applying algorithms and computational models to the trove— could uncover patterns that would help the organization operate more efficiently.
He brought it up at a staff meeting. The response was enthusiastic, but then came the inevitable question: How can we afford to hire someone to do it?
“We are teaching students how to think like productive citizens.”
“I said, well, I know a college,” Spinosa joked.
He pitched the idea to Emily Wiley, associate dean of the faculty and professor of biology at CMC. They’d met about a decade ago, when Spinosa had arranged a talk on genomics at the Marian Miner Cook Athenaeum, and stayed in touch. Wiley was helping to develop CMC’s new data science sequence, which included a capstone project for students, and thought Spinosa’s proposal might be a great fit. Her hunch was spot on.
That conversation evolved into an interdisciplinary team of students conducting a data science pilot project last spring. Under the guidance of Wiley and Jeho Park, visiting assistant professor and director of the Murty Sunak Quantitative and Computing Lab, the students mined the SDBB’s data for information that would help the nonprofit make the best use of its resources.
For Wiley, the pilot was an example of the interdisciplinary, collaborative learning that she has championed throughout her own career—and that is a distinctive strength of CMC. As scientific research increasingly stretches across traditional disciplines such as biology, chemistry, and physics, projects such as this—team-taught, gathering students with diverse interests and skills—are necessary preparation for students heading into careers in science and medicine. Further, proficiency in a broad array of quantitative skills is crucial for all students as technology continues to transform industry, business, and our daily lives.
“This project illustrated to us that we can bring together students from different majors to work together collaboratively and productively. We can break really big questions into smaller, solvable parts, divide up tasks, conduct research, analyze and poke holes in findings, and then present them—the whole scientific process,” said Wiley. “We are teaching students how to think like productive citizens.”
A multifaceted team
Kelly Watanabe ’20 wanted in as soon as she heard the word “interdisciplinary.” In high school in Honolulu, Watanabe had hated physics and expected to major in biology at CMC. A first-year class that combined biology, chemistry, and physics changed her mind. “I saw how the areas of biology and physics illuminated each other, and I became a biophysics major,” said Watanabe. “I think there is a lot of value and new things to be learned when you combine disciplines, and I also like when there are multiple professors so you learn from their different perspectives.” Working with a blood bank was particularly appealing to her, as the project would integrate biology and quantitative research. “This was a good opportunity to take some of the skills I’d learned in biophysics and math modeling and apply it to data science and interpretation,” said Watanabe, who is a long and triple jumper for CMS women’s track and field. “One of the most valuable things anyone can learn is how to tell a story from data.” In data science, that story is both descriptive—understanding what the data actually reveals and looking for patterns within it—and inferential—using computer modeling to predict what the data suggests might happen in the future.
“One of the most valuable things anyone can learn is how to tell a story from data.”
The pilot was a “perfect fit” for Naveen Shastri ’20, a computer science and economics major from Northern California. “Economics is about understanding specific models, such as supply and demand, but with computer science you design specific programs to answer specific questions. I learned how to be creative in CS.” Shastri is pursuing a career in finance, and wanted to participate as the project focused on an organization’s actual challenges. That it had a social mission was a bonus.
Park and Wiley rounded out the team with two students with strong coding ability: CMC’s James Ren ’19, a computer science major, and Lathan Liou ’19, a Pomona College pre-med. On the first of what became weekly calls, the team’s new client described the students’ overarching goal: to discover which factors were associated with collecting the most blood, and the most needed type of blood.
Like all blood banks, the SDBB has a complicated task. Volunteers donate blood at one of its six locations or during a blood drive— its 12 bloodmobiles cover a wide swath of Southern California. But running blood drives is not an exact science. The number of donors participating varies widely and is difficult to predict. Of those who do sign-up, about 16 percent either don’t show or don’t wind up donating during the event.
Also hard to estimate: the characteristics of the blood that is collected during each drive. There are four primary blood types—A, B, AB, and O, which is the most common, and therefore, the most needed by hospitals. Those groups are only the beginning. Blood banks must separate and sort all donations by 32 factors, creating distinct products for patients. “If someone needs a transfusion, you have to find blood that matches their group and with the right antibodies,” said Spinosa. “It’s a time-consuming process.”
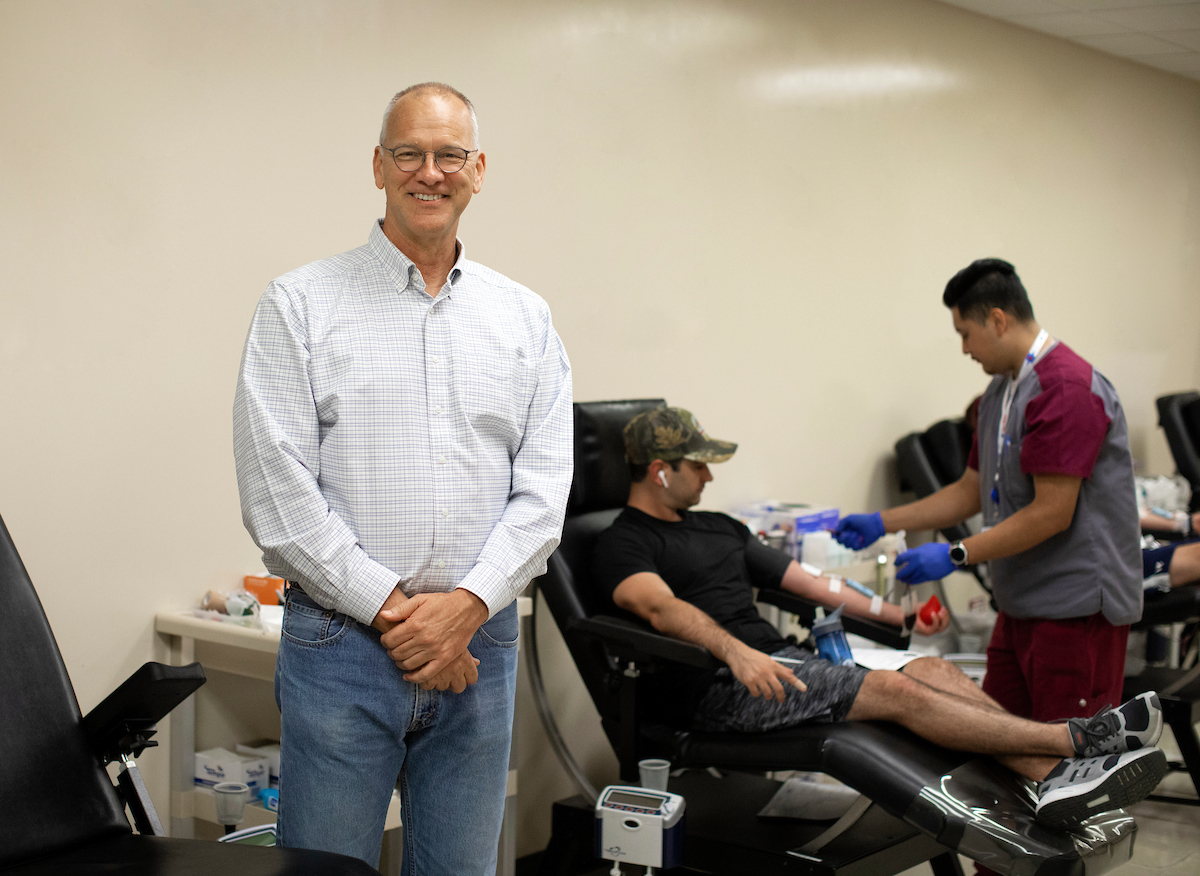
Working together
As the students began to understand the SDBB’s work and the questions it wanted answered, they divvied up tasks. “More than each having a deep knowledge in an area, what we saw was that they had different soft skills and played to those strengths,” said Wiley. Shastri agreed that the team’s mix of skills improved the experience. “We talked about how to complement and help each other,” he said.
The first challenge was getting a handle on the data set itself, understanding what information had been collected, and making sure it was consistently categorized and labeled. Once over that hurdle, the students refined the SDBB’s broad mandate so they could develop computational models to answer specific questions. “Because it was a pilot, and because they asked us for ideas, it allowed us to look for our own ways to solve the problem,” said Shastri. “That allowed for creativity.” Professors Park and Wiley were always available to the students, but also wanted to give them a lot of leeway. “That was perfect because it taught us to work together to decide how we wanted to learn, rather than being told,” said Shastri.
Watanabe said she wasn’t as strong on computer skills as the rest of the team, but developed those with her teammate’s assistance while keeping the project organized and on track. Shastri’s role was to look at donor trends in specific types of locations. At first, he struggled. Shastri wasn’t sure which type of modeling would reveal the most information about a location. Clarity came after he visited the blood bank, where talking to staff and seeing how they spent their time helped put the data into context. After trying several models, Shastri developed a logistics regression model that looked at two populations: Millennials, a group the blood bank wanted to court, and donors with the O blood type.
In addition to Shastri’s model, the team used a linear regression model to identify variables that might predict the amount of blood donated, a time series model to identify trends in donations over time, and a decision tree framework to help predict whether a donor will complete the donation process.
“When you are working with large data sets, the questions can morph as you get going, which is exciting,” said Wiley. “But learning to communicate back and forth with the team and the client as things evolve is important.” Another benefit of applying the scientific method for every student: developing patience. “Much of their lives now revolve around getting instant answers, so it is good for them to keep testing and questioning,” Wiley said.
After the team presented its research to Spinosa on campus in May, he deemed the pilot a success. The SDBB can build on the students’ work to help guide decisions as it moves forward. More important, he said, was seeing the students successfully collaborate to tackle problems.
“I am quite enamored of working with CMC students,” Spinosa said. “This kind of real-world experience is exactly what they need to be prepared to succeed when they graduate.”